Insurers: the rise of partnerships with AI specialist vendors
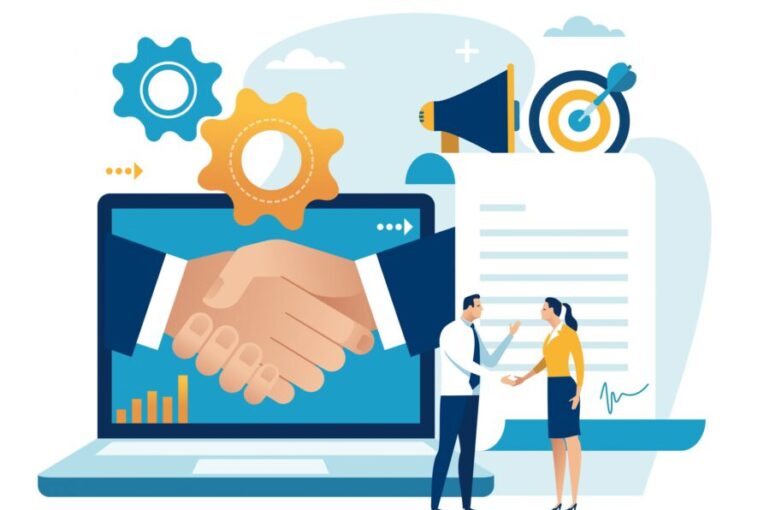
We are in 2021, and insurance carriers are now fully aware that Artificial Intelligence is a key component for standing out and rising above competitors like big techs and fullstack insurtechs. It’s not a surprise that according to a recent survey published by KPMG, financial services is where the rate of AI adoption is the highest among all industries (+37% in one year).
Under pressure, incumbents are preparing radical changes to their IT infrastructures, to move legacy systems to the cloud, and place data analytics, machine learning and AI at the center of their organization. In this unprecedented effort to ultimately improve customer experience, insurers are massively investing to develop future-proof AI capabilities like natural language processing, computer vision or speech analytics.
One aspect of this transformation is the extensive hiring of new generations of talents such as data scientists, machine learning engineers, data software engineers, data architects, etc. …, despite the extreme difficulty of building such teams, due to the scarcity of these skills and organizational complexities.
Another perspective of this revolution is the launching of dozens of internal innovative AI experiments all along the insurance value chain: claim management, customer service, sales, underwriting, fraud, etc…. – for all types of business lines (health, P&C, life, …) – and all categories of policyholders (personal, commercial, …).
The new legacy
And that’s where complications arise. First, the combination of potential AI projects to launch is too huge for insurance carriers to address all of them alone: it represents a jungle of sometimes 50, 80, or even 100+ candidates. Order of magnitude is higher than what most organizations can tackle internally, due to the bottleneck of available resources. Prioritizing is hard. Which projects to select first? Which ones will drive the biggest Return on Investment (ROI)? How to measure an AI-driven project ROI without too much uncertainty?
Second, even if launched, most of these innovations remain at the stage of Proof of Concepts (PoCs). Very few go to production, letting investments left without any ROI. And for the rare experiments that go live, it’s only the beginning: they have high maintenance costs, often higher than the design costs themselves. Indeed, AI-first applications are not like traditional software: machine learning models have to be regularly retrained and data drifts have to be carefully monitored.
What’s more, an additional complexity comes from the necessity for AI algorithms applied in financial services to comply with the coming regulations to guarantee their governance, ethics and fairness.
Finally, if it was not enough, the underlying AI algorithms and technologies have also to be updated regularly to remain up to date. Look for example at NLP: in this discipline only, state of the art is evolving every 3 ou 6 months.
As a consequence, we understand that in the AI race, there is a high risk for insurers to exhaust their organizations with custom AI projects that remain undeployed in production – or if in production, that need such an amount of sophisticated and costly maintenance that they will not be able to cope with. All this becomes, in a way, a new technical debt, a new generation of IT legacy.
Mitigating the risk of a new legacy by partnering with insurance specialized AI vendors
For very experimental AI usage, not clearly defined, or very specific cases where no off-the-shelf solution exists on the market, it makes sense for incumbents to launch proprietary developments. But for some very common and carefully selected AI usages, instead of creating from scratch new in-house solutions, and being exposed to the risk of adding again a new legacy to the old legacy, an obvious and efficient solution for incumbents is to partner with specialized off-the-shelf tech vendors (like for example Zelros regarding advanced distribution topics, or other specialist vendors for other areas like fraud detection, risk prediction, claim automation, and so on).
The first reason is that it ensures that there is a case with high and measurable ROI. Indeed by design, insurance specialized vendors focus on AI usages that are mature, reproducible, and with a viable business model.
Second, it’s easy to get references from existing users, to validate beforehand the value of the solution. It helps secure the investment, and guarantee its success.
Finally, it allows a faster go-to-market: deployments within weeks – because the technology is already available – instead of months or years when developed internally. This is crucial for insurers to avoid being left behind and losing in competitive advance. What’s more, partnering with a vendor safeguards an access to regular technology updates and new state-of-the-art capabilities, while controlling the Total Cost of Ownership (TCO). For instance Zelros releases new innovative platform features three times a year.
Consider developing internally the AI solution | Consider partnering with insurance specialized AI vendors | |
You lack resources like data scientists, machine learning engineers, etc. … | X | |
Your project is not well defined or experimental | X | |
Time to market, or ROI are at stake | X | |
Your project is very specific, there is no similar case out there in the market | X | |
You have many AI projects in parallel, and have more demand than what you can deliver | X |
Questions incumbents should ask to decide whether to partner with an insurance specialized AI vendor
You got the point: this is not surprising that incumbents are more and more willing to partner with insurance specialized AI vendors. And it’s just the beginning. GlobalData, a data and analytics consulting firm, forecasts that the insurance sector will accelerate investments in AI software in the coming years, reaching $3.4bn in 2024 from $1.2bn in 2019, representing a huge compound annual growth rate (CAGR) of 23% over this period.
