Inventing the Financial Services Workspace of the Future!
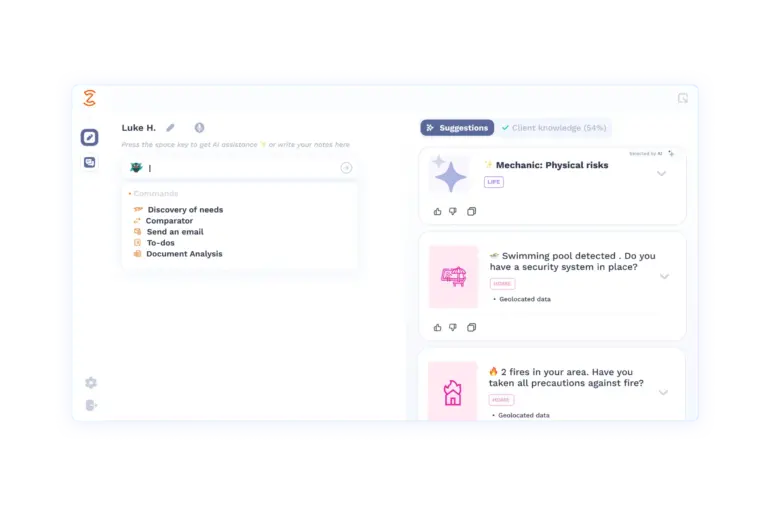
Introduction
It may seem like we’re speaking as veterans, but the reality is we began our careers just as the mainframe era was ending. The financial services industry has always been a heavy user of mainframes, and it continues to rely on them. Leading financial institutions once provided their employees with command-line black screens as they transitioned from paper-based to computer-based operations.
Then came the era of click-button-rich interfaces, with horizontal players like Oracle and Salesforce and verticalized players like FIS Global or Guidewire.
So, what’s next? At Zelros, we are on a mission to create the Financial Services workspace of the Future, and we couldn’t be more excited. 2 recent studies, estimates the impact of generative AI applied this industry : Citi Global Perspectives and Solution study says AI Could Add $170 billion or 9% to Global Banks Sector Profit Pool by 2028 while Bain & Company study says it could yield over $50 billion in annual economic benefits to insurance distribution alone.
Where will the biggest breakthroughs come from? We are eager to share our vision for the future of financial services desktops, contributing to the driving forces that will accelerate change. All of this will ultimately benefit employees and clients.
We’ve outlined a four-step roadmap to the future, from low-hanging fruit to the most advanced changes. You might see these as parallel paths bringing new value to your employees and clients.
Let’s be clear: we’re not talking about a 30-year journey. The pace of change is rapid. What we describe here is more of a 5 to 10-year journey. Don’t miss the train!
Step 1: Get a client 360° view – for real!
Many organizations have faced challenges due to being misled by software vendors, resulting in a ripple effect where employees and their clients are also misinformed. Even with the rise of AI, genAI, predictive AI, and personalization technology in the financial services sector, it appears that customers are yet to receive the level of service they truly deserve. The truth is, a true client 360° view, that gathers all the meaningful and latest information from every possible source, is a daunting task, costly and time consuming if not done right. Global protection gap of US$900 billion for cyber and US$100 billion for natural catastrophes*!
At Zelros, we believe that one of the greatest values insurers will gain from large language models (LLMs) is their ability to bridge the gap between two worlds that have historically existed in silos:
- Structured Data Sources: Data properly stored in business systems such as CRM, Core Banking, Policy Management Systems, Claims Systems, and more.
- Unstructured Data Sources: Information found in knowledge bases, emails, documents, voice discussions, and other less organized formats.
By integrating these disparate sources, LLMs can provide a more comprehensive and insightful view of clients, enabling insurers to make better-informed decisions.
Up until now, employees have relied on their own expertise to bridge the gap between these two worlds. With LLMs, it is now possible to provide employees and clients with a holistic view that leverages both structured and unstructured data. This is a significant game-changer, as it enables the automation of decision-making processes that were previously handled entirely by human brains.
To give an example, from an LLM perspective, having a structured machine-generated record (e.g., from a CRM) as input is no different than having an unstructured record like for example a voice call transcript or raw notes taken by an agent during a needs discovery interview:
CRM structured data | Voice call transcript | Advisor notes |
{“birth_date”: “1984-03-03”,“address”: “4537 Maple Drive Sunnydale, CA 94102 United States”,“marital_status”: “married”,…} | “I’m contacting you to know because I would like to buy a cat for my daughter, and I would like to know if you provide pet insurance, ….”or raw notes taken by an agent during a needs discovery interview“ | “- has 2 children- wants to change car in the 2 coming years- owns a 3-room apartment insured by Insurer X“ |
In addition, LLMs enable the reconciliation of data from different heterogeneous systems. For instance, they can integrate information from a P&C core system A and a Life core system B. This capability arises from foundation models that understand IT systems, such as recognizing that fields named date_of_birth, birthDate, BDate, or DOB across various insurance core systems all represent the same information.
At Zelros, our platform is designed to deliver decisions that make the most of any relevant data sources, whether structured or unstructured. Moreover, our platform increasingly leverages both internal and external data sources to provide a comprehensive view tailored to each customer’s context.
Step 2: Forget about clicking buttons or keying in information; let your machine listen instead.
Think about it: we don’t create any value by clicking buttons or keying in information. The goal is simply to save information electronically for future use. We’ve been doing this for decades because we need to store data properly in a tabular form for analysis, comparison, and processing.
The good news is that your machine can now listen to your speech, transcribe it into full text, intelligently extract all important information, and store it in the proper place as before.
Imagine the productivity gains and improvements in data quality. Employees will save time, clients will find the process user-friendly, and you’ll capture valuable client knowledge that was previously lost. This knowledge can significantly enhance your engagement strategy.
Clients share a wealth of information, both inadvertently and willingly: their hobbies, dislikes, vacation spots, pets’ names, car buying processes, future project desires, expectations, fears, and more. All this information presents tremendous opportunities to better serve them according to their financial goals and protection needs. Don’t wait to delight them!
Step 3: Your machine can and will do more. It’s up to you to co-work with your Copilot.
We cannot emphasize this enough: bank and insurance products are complex, and clients often have low financial literacy levels both in banking and insurance products. Adding to this complexity is a significant amount of regulation. The consequence is that most decision-making has relied heavily on the expertise of specialized employees: advisors or agents, actuaries, marketers, underwriters, risk managers, claim handlers, and so on. If any industry epitomizes the knowledge worker sector, it’s the financial industry.
Traditionally, tools have mirrored business organizations: segregated and siloed. Now, with machines capable of going further in assisting employees and with clients demanding smoother experiences, we see the lines beginning to blur. Insurance agents, for example, have expanded their expertise, dabbling in underwriting, customer service, and claim management.
With the advent of smarter decision support systems, this trend will accelerate. No longer should three different people manage three different tasks. Instead, an specialized Copilot can guide and coach a single individual to handle a wide array of tasks, reserving experts for edge cases. What was once impossible to fit into a single human brain can now be seamlessly integrated into a machine brain, broadening the scope of tasks a single insurance employee can handle.
Beyond massive productivity gains, the most significant benefit is greatly improved customer experiences, eliminating the loss in translation and ensuring smoother interactions.
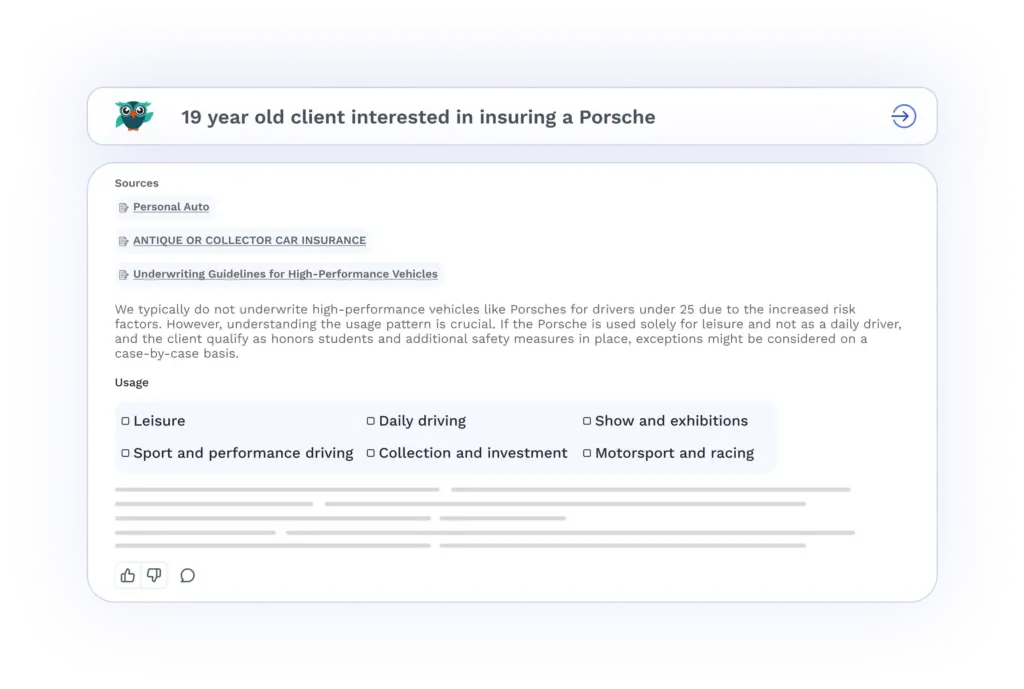
Step 4: Reskill and refocus – Autopilot is around the corner
What’s the difference between Copilot and Autopilot?
Level of trust and autonomy. It’s only a matter of time before you’ll find your Copilot expert and reliable enough to handle some tasks independently. At Zelros, we are already paving the way for Step 4, where autonomous virtual agents make decisions based on LLMs.
We are building a suite of agents with predefined sets of skills and tools that can autonomously orchestrate complex tasks while interacting with users to achieve superior goals.
For example, here is an individual LLM agent expert in comparing legal protection insurances.
Does this sound like something straight out of a science fiction movie? Well, it’s not. It works. While it’s not yet in large-scale production for many scenarios or use cases, it is effective. We kindly advise you to start planning how to reskill and refocus your human resources. Relax—there are plenty of opportunities to create value in areas that won’t be touched by autopilots.
Conclusion
Inventing the future of the financial service workspace is all about shaping the future of work in bank and insurance. At Zelros, we have been enhancing existing applications with AI decisions in this industry for the past eight years. If you want to know more about how we shape this future of work, have a look to our summer release Amber Wave 🏄 paving the way for the advent of Agentic AI
*The Global Federation of Insurance Associations (GFIA) said in a new report.