5 benefits of a MLOps culture
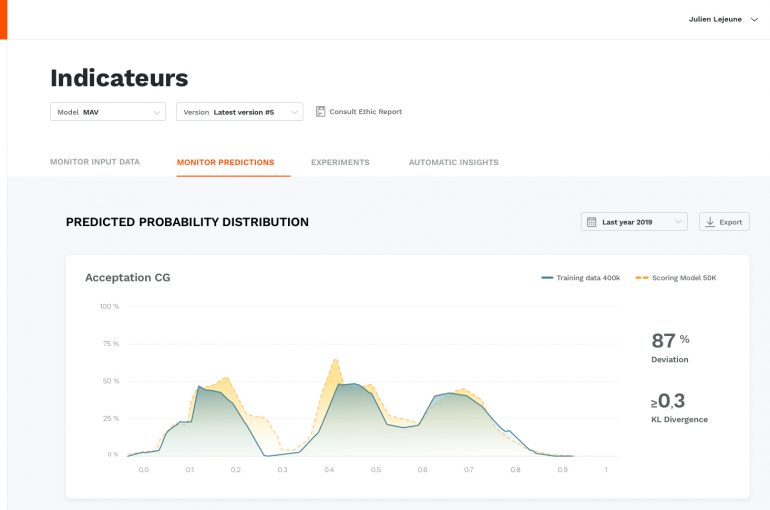
By Johana Moreno, Product Owner
Curious to know more? Contact us or meet us at ITC Las Vegas at booth 3253!
The insurance industry has ushered in a new digital era in which customer experience and preferred interaction is being converted to digital, customers want their problems solved quickly, and new generations prefer services with a rich digital experience.
Insurers are looking for more automation (beyond document automation) and intelligent technologies to address all these trends, which have been exacerbated by the Covid crisis. This is where AI fills the gap and occupies a large space for automation and services with exceptional customer experience.
As an AI company, Zelros has been successfully using its machine learning models for various customers, and a wide range of applications in the insurance industry overcoming many challenges. On the one hand, implementing accountable tools to demonstrate the positive impact of our AI models for very demanding customers, and on the other, providing the gold service and reducing time to market. These have allowed Zelros to reinvent and optimize its process without compromising quality.
Machine learning is not an insignificant task, behind the scenes all our teams need an important organization to bring high-quality models to production. It requires the combination of two skills Data Science and Devops. AI software editors are facing difficulties to deliver and enhance AI solutions. Actions like data cleaning, data collecting, model training and validation, model deployment, and retraining are most of the time performed manually. This can mislead to operational errors and impacts on productivity and business performance.
At Zelros, we believe that culture and environment based on ML technology can bring high business value. Ensuring clear governance for AI lifecycle processes and good automation technology contribute to a robust, transparent, and trustworthy AI.
To respond to these challenges, the Zelros platform provides a sustainable cycle for delivering ML into production, a way to orchestrate Data scientists and system integrators activities to work better together, to gain customer confidence and to found our AI solution on transparency and fairness AI principles.
What are the benefits of using our MLOps platform in Insurance?
1: Reduce time on data collection and data preparation
Data Scientists, systems integrators and solution engineers used to spend a lot of time with repetitive data acquisition or data preprocessing tasks before they could get their hands on the model and use our use cases. However, these tasks were fastidious and costly as many highly skilled resources were allocated before the model was built. MLOps can widely benefit data scientists and software engineers to reduce these operational tasks.
We wanted to reduce time connecting customers’ data to Zelros AI platform and to be able to leverage all use cases with fast data connectors. Obtaining up-to-date data is the most important thing to provide a powerful algorithm. For this reason, we paid special attention to data normalization, building and creating a standardized data model that would speed up the deployment process.
- Normalize data: A standard model is a data architecture where the data is stored, and customers can provide and add information that fits the AI use case. This normalized data provides the capability to use a centralized data environment with all features in one place rather than merging files and overheads from all different data sources every time a new feature is implemented and repeating it for each client. Data scientists can now work on one centralized data environment that respects data protection and data handling policies.
- Ensure Accuracy: Zelros guides its system in a process cycle in which data is regularly updated, allowing AI to evolve with up-to-date data to ensure the AI model’s response to the behavior and representation of the last population. Data scientists don’t worry anymore about updating data and focus only on model performance.
2: Automate Model Building (Ready to use)
After data scientists and system integrators collected and cleaned up data, they had to manually create, validate and deploy the model. These actions could mislead to errors and lead to overrun in operational costs.
To truly create efficiency in operational tasks, training and deployment pipelines need to be automated. Automation can benefit Data scientists to focus on what they do best, extracting business-focused insights, research and looking for innovation and revolutionary techniques to solve AI Ethics issues.
The lack of automation was one of the main difficulties; we transformed our traditional pipelines into an AutoML pipeline where our data scientist can simply select the use case and generate a specialized insurance model in a click. This fully automated pipeline continuously trains models resulting in a ready-to-use API.
Most of our customers had a long lifecycle for updating their software with the difficulty of upgrading their legacy systems. Besides, every client use case is unique, and the way models’ predictions are used can differ from customer to customer (we do not use data from one client to another). To facilitate interconnections between clients and our platform, Zelros supplies an API collection included on Zelros MLOps automatization pipeline, allowing us to cut the deployment time from 4 to 2 months.
3: Accelerate the validation process
The biggest AI lifecycle challenge is to scale from a small project to a large production system. To move forward, validation tools and transparency are key in the decision-making process, which sometimes requires validation from the business to the legal department. Stakeholders must be able to rely on measurable information before taking the big step.
Responsible AI is one of the greatest concerns at Zelros and we pay big attention to this principle. AI automation approach also applies to documentation, and Zelros MLops pipeline includes an Ethical and Fairness report, detailing the AI model in terms of processing, input data, prediction, completeness, behaviors, and other statistical metrics.
With a plurality of stakeholders on AI projects, automatic reporting has demonstrated its advantages, such as communication and validation facilitator. The insurance and finance industries are very regulated sectors where decisions made by AI algorithms need to be transparent and follow a strict process. Reporting can facilitate the work between Insurers and external regulators like ACPR or BaFin. For example, documentation can help to support the task of auditing, validating data processing, challenging models, explaining global and local decisions, benchmarking results and tracking compliance tests.
Furthermore, automated reporting helps to understand the client’s data and select model candidates to go for production. Providing enough documentation to explain the AI and its limitations can contribute to building trust in AI.
4: Monitor and Re-train
Deployment of AI into production is not the last step on an AI project. Without a doubt, to put into production at scale is a big achievement, but our work is not over.
As an AI company, we must ensure that we cover all phases of the AI life cycle. The traceability of a model through production is part of Transparency and Trustworthy AI principles. For this reason, monitoring is one of the most crucial parts of deploying a model. It is important to continually track behavior and performance and anticipate drifts before consequences occur on the business side.
Monitoring the health of a model on production is a task that requires special care. It enables data scientists and other team members to monitor model decays, drifts, outliers, bias, and have a proactive approach. For this purpose, a visualization tool where data scientists can observe, analyze during the time, understand, and detect anomalies are a key for maintenance. Our goal is to provide insights from real-life data to prevent the model from serving bad predictions.
5: Experiment with real data
We have explained why MLOps is significant to the Zelros AI platform at every stage of the AI lifecycle, from data collection to monitoring abilities. However, AI’s permanent life relies on our capacity to improve AI models during the time. Zelros latest inventions and researches contribute to sustaining our AI models, but there are some questions to solve for AI preservation:
- Why are stakeholders going to accept the new AI model version if the current version is working?
- How to ensure stakeholders that the new model will not introduce anomalies?
- How to give the right metrics to compare model version performances?
These questions should not be underrated. At Zelros, we know how important it is to secure and minimize risk for our customers.
Providing elements that can contribute to the feedback loop is important, just as conducting model experiments with real data appears to be a good solution at first glance.
Shadowing or A/B testing techniques are a good example of how Zelros can handle model experiments, so customers can choose their testing strategy. Experiments can be a helpful tool to comfort or confirm the decision making for generalization. Their main advantage is that real data can be used to assess the quality of predictions, and also a way to compare the results between models.
Conclusion
Delivering an AI model on production means more than trying to get a performed mathematical algorithm that will produce high ROI and business impact. At Zelros, we are committed to producing Responsible AI at every stage of the AI lifecycle. Designing an MLOps workflow, not only benefits in terms of automatization, costs and productivity optimization. It also allows establishing good practices for a Transparent, Explainable and Trustworthy AI, ensuring that all insurance and finance algorithms respect AI model governance policies.