Why Automation is the Next Frontier of Enterprise AI
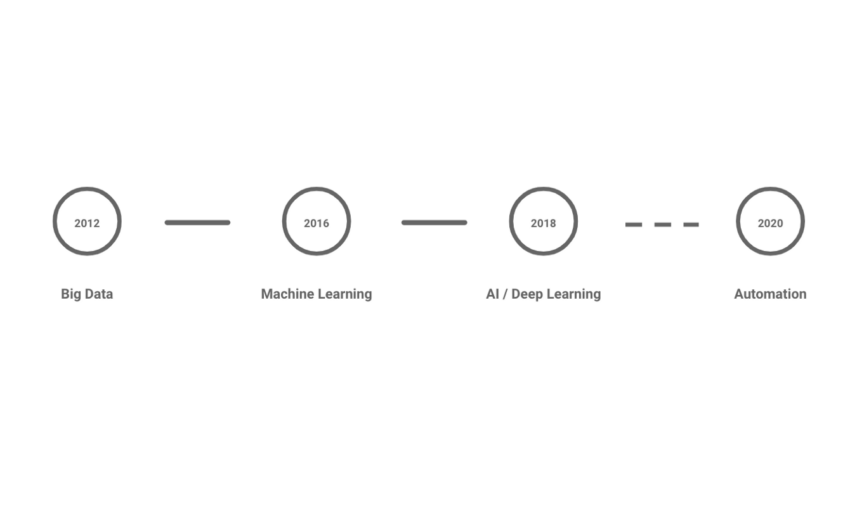
A short history of enterprise usage of Data
When you look into the past, you realize that the vocabulary used to describe the intelligent usage of data has evolved a lot.
Everything started in 2012, with the term Big Data. Remember? It was when the planet understood that our society was going to generate gigantic amounts of data, due to smartphone usage, cloud apps, IoT, etc. … and something useful could maybe be done with it.
If you think about the essence of the name Big Data, it was about just collecting raw data, but not yet about processing it — and even less about knowing exactly for what purpose to use it. There was an intuition that something was happening with data, but nothing was concrete and really mature at this time.
Today Big Data is not used so much. Look at its google searches: after a huge initial growth, is has plateaued and seems to even start decreasing.
The name Big Data was about just collecting raw data, but not yet about processing it — and even less about knowing exactly for what purpose to use it
The raise of Machine Learning
So what term came after Big Data, and made it old fashioned? Machine Learning. This expression exploded in 2016, and quickly exceeded Big Data in 2017.
The word Machine Learning is interesting, because for the first time, we were talking about the intelligent processing itself, of collected data. We started to do something tangible with data: predictions, recommendations, forecasts, decisions, etc. …
The art of using data in a smart way took an other incarnation with the term Data Science (and its associated job: data scientist, called at this time ‘the sexiest job of the 21st century’ ?), from which Machine Learning is a category.
But still Data Science and Machine Learning remain tech expressions, lacking clear business usage or outcome behind them.
The word Machine Learning is interesting, because for the first time, we were talking about the intelligent processing itself, of collected data.
AI and the use of data in end-to-end systems
Then came the rebirth of Artificial Intelligence. This expression has been very popular 20 years ago (and even more in the ’90s), then has been forgotten between 2008 and 2016, and finally became fashionable again.
Now with Artificial Intelligence, we started to illustrate that data, and the fact of processing it with machine learning, could be used in complex systems to build a new generation of enterprise software. This was applicable on all types of industries: automotive, retail, finance, energy, education, … and all type of data: images, speech, documents, …
What’s more, the purposes and outcomes started to be better defined: improve an HR hiring process, increase a commercial sales efficiency, optimize a logistic network, …
However, there has been probably too much enthusiasm around Artificial Intelligence (and its incorrectly associated equivalent Deep Learning). Yes it could solve a lot of problems, but not all problems, and actually the problems really worth to be solved with AI / machine learning / data are not easy to spot.
As a consequence, since a few months we start to observe an AI and deep learning fatigue, clearly visible on the graph below. While data science is still getting traction, showing that the usage of data itself is not questioned, and still future proof.

Since a few months, we start to observe an AI and deep learning fatigue
Using AI to automate enterprise processes
So what’s next after AI? We believe that automation is a good candidate to describe the next stage in enterprise usage of AI.
Indeed, when an AI system fully automates an enterprise process at scale (like e.g. automatically handling insurance subscriptions, without any human intervention) — as opposed to when it is not used to directly automate something (like e.g. monthly forecasting a logistic demand) — it must embed extra design attributes that make it actually more mature. This AI maturity, hard to achieve, can be illustrated by at least four characteristics: robustness, ethics & transparency, economic viability, and entry barrier.
When an AI system fully automates an enterprise process at scale, it must embed extra design attributes that make it actually more mature than others.
Robustness
By definition, the underlying predictive system used in an AI-automated industrial process must be 100% robust — especially as there is no human in the loop. Indeed, you can’t rely on it, if there is a risk of instability, or if it is not designed to be antifragile.
Ethics and transparency
When automated decisions are taken thousands and thousands of time per day, you must be sure that what you are doing is fair, and allowed by the regulation. If it is not, chances are low that your AI gets automating anything, and has real impact.
Economic viability
Using AI to automate a process has a cost, coming generally from software and/or computing ressources subscription. The benefit of this automation must be significantly higher — 5X to 10X — than this cost, to get a clear ROI (Return On Investment). The outcome can be for example additional revenues, or an increased operational efficiency.
In this sense, the economic viability of an AI automated process has to exist. Put in an other way, even the fanciest predictive system, but without any demonstrated ROI, won’t be used to automate anything in the long run.
Entry barrier
Finally, to be able to exist, an AI system automating a process must have a certain level of necessary complexity, not reachable with a trivial alternative. Indeed, if the process can be automated by simple decisions rules (eg.: if age > 30, then …), and no — or weak — AI is needed, this is not where AI automation will be sustainable. A traditional software — or RPA: Robotic Process Automation, ie. hard coded decision rules — will just do the job.
See you in 3 years ?
Let’s have a final look at the trend of the word automation. After 10 years of decrease, it seems to increase again. What will happen next? Let’s meet in 3 years to see what automation has become, and what is the next big trend!